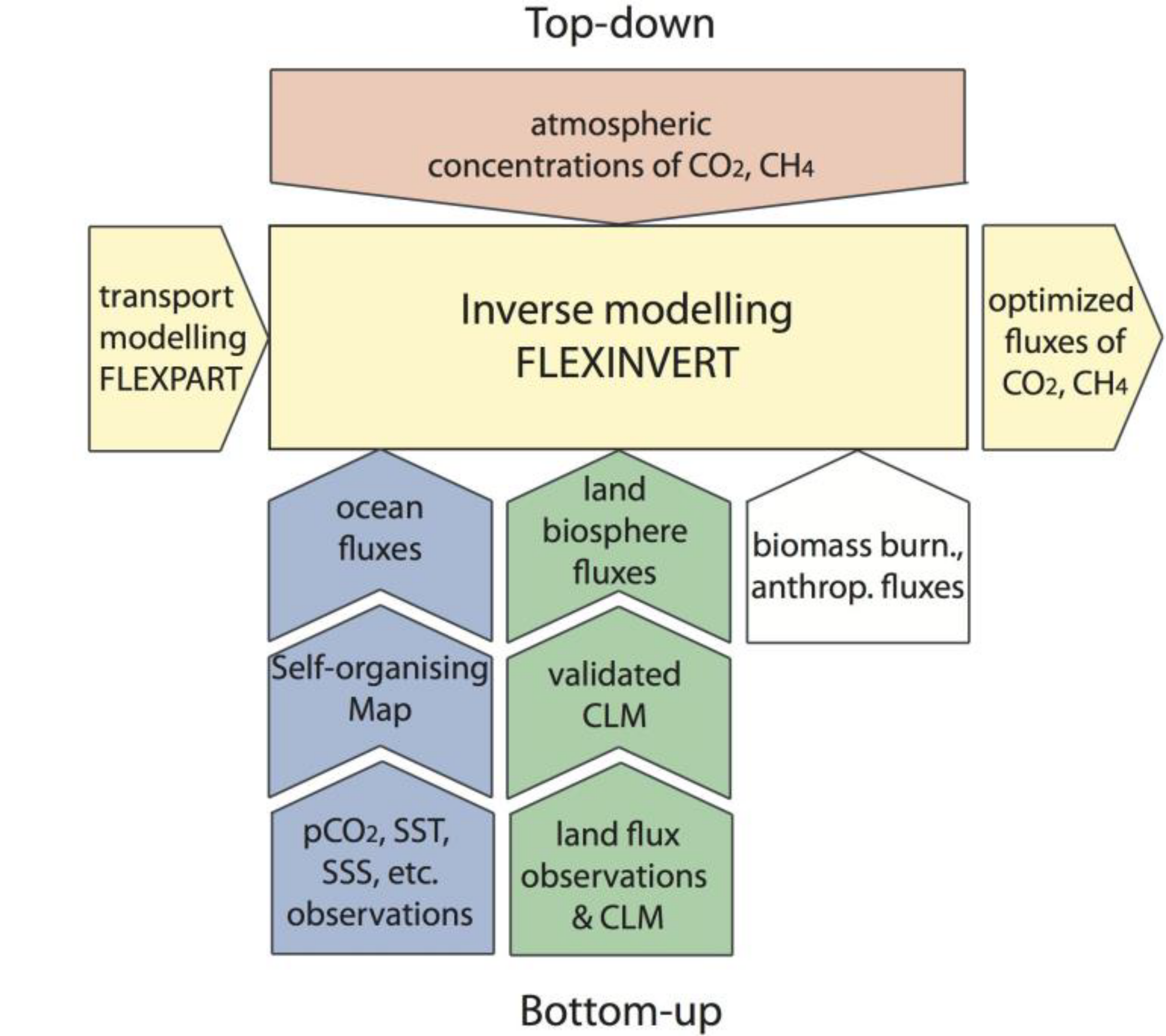
The motivation for ICOS Norway to collect carbon data is to improve our understanding of the regional carbon budget. However, it is by no means trivial to obtain spatially and temporally resolved ocean and land fluxes and to attribute these to biogenic and anthropogenic components. The measurements alone do not provide this information and, thus, an integrating effort is needed that utilizes all data obtained to provide a best estimate of Nordic and European carbon fluxes. The figure above illustrates the integration effort of the ICOS Norway consortium.
At the heart of the integration effort is a method called inverse modelling, which is a statistical optimization technique that merges information from different sources. For this, NILU has developed an open-source atmospheric inverse modelling framework called FLEXINVERT that optimizes greenhouse gas fluxes to optimally fit with observed concentrations (Stohl et al., 2009; Thompson and Stohl, 2014). The spatial resolution of the fluxes can be varied and is partly determined by the geometry of the observing network.
To utilize the atmospheric concentration measurements of greenhouse gases, the inversion requires a link between greenhouse gas fluxes and their concentrations, so-called source receptor relationships. These are taken from an atmospheric transport model. FLEXINVERT utilizes NILU’s Lagrangian transport model, FLEXPART (Stohl et al., 1998; 2005), which has become one of the most widely used atmospheric models worldwide. The advantage of this model is its capability to provide high spatial resolution, especially around receptor sites.
The observations alone do not provide sufficient information to directly determine the fluxes of CH4 and CO2. Therefore, the inversion requires additional input information, so-called a priori fluxes. The a priori fluxes are based on best information of the greenhouse gas fluxes that is available from emission inventories, land surface models, mapping of the pCO2 distribution in the ocean, etc. The inversion is a statistical optimization technique that adjusts these a priori fluxes in a way that the modelled concentrations agree optimally with the observed concentrations, while penalizing unrealistically large deviations from the a priori. The coarse resolution of the ICOS observation network in the Nordic countries and the complex shape of the Norwegian territory will limit our capabilities of improving flux estimates separately for Norway and other Nordic countries. This separation will therefore depend to a large extent on a priori information.
Ocean fluxes: To provide ocean a priori fluxes of CO2, the pCO2 distribution in the Nordic Seas and northern North Atlantic are mapped using a neural network approach. Specifically, we adopt the Self-Organizing Map method that has been used previously in the region, with great success (Kvæstad, 2013). This approach uses variability in sea surface temperature (SST), sea surface salinity (SSS), chlorophyll, and mixed layer depth to represent surface ocean pCO2 variations through a trained and labeled neural network.
Terrestrial fluxes: For the prior estimate of fossil fuel emissions, we use information from emission inventories such as EDGAR or ECLIPSE as provided in emission data bases. For biomass burning emissions of CO2, we use estimates from the GFED database. Prior information on the terrestrial biosphere fluxes is taken from the Community Land Model (CLM) that is run at CICERO Centre for International Climate Research (CICERO).
Inverse modelling: All flux data sets described above will be utilized by NILU as a priori information in the inversion. FLEXINVERT will use the temporally resolved atmospheric concentrations of CH4 and CO2 from Birkenes and Zeppelin as well as other ICOS stations and flask- sampling sites in Europe, and the output of the FLEXPART model, to derive improved a posteriori fluxes. The focus will be on optimizing the terrestrial biosphere fluxes, since they have the largest a priori uncertainties. For CH4, we will constrain the total emission (from wetlands, biomass burning and anthropogenic sources) and provide temporally and spatially resolved emission maps, in analogy to a recent study with a focus on high-latitude CH4 sources (Thompson et al., 2016).
Validation: Terrestrial biosphere flux data from ICOS-Norway, and other Scandinavian flux measurement sites, will be used by NIBIO and CICERO to evaluate the carbon fluxes provided by CLM for the land use types that are particularly important in Fennoscandia. NIBIO will furthermore conduct advanced plot-scale modelling of the carbon cycle and GHG fluxes for the relevant Nordic stations using different ecosystem models. For grid cells in Fennoscandia where flux measurement sites are located, NILU and NIBIO will also compare the inversion-derived a posteriori and observed fluxes.
Collaboration: The national integration will be led by NILU in close co-operation with the UoB, NIBIO, and CICERO. The key deliverable will be national flux data sets to the national user community/group following as closely as possible the national policy requirements - however, considering the constraints due to the coarse measurement network. In addition, we will produce European flux maps in close collaboration with other European ICOS groups, and we will support the European model ensemble activities with the Norwegian inverse model, FLEXINVERT.
Contact person: Christine Groot Zwaaftink (cgz@nilu.no)
References
Kvæstad, E. (2013), Mapping the fugacity of CO2 in the Nordic Seas and the northern North Atlantic Ocean, MSc thesis, Geophysical Institute, University of Bergen.
Stohl, A. et al. (1998), Validation of the Lagrangian particle dispersion model FLEXPART against large-scale tracer experiment data, Atmos. Environ., 32 (24), 4245-4264.
Stohl, A. et al. (2005), Technical note: The Lagrangian particle dispersion model FLEXPART version 6.2, Atmos. Chem. Phys., 5(9), 2461-2474.
Stohl, A. et al. (2009), An analytical inversion method for determining regional and global emissions of greenhouse gases: Sensitivity studies and application to halocarbons, Atmos. Chem. Phys., 9 (5), 1597-1620.
Thompson, R.L. and A. Stohl (2014), FLEXINVERT: an atmospheric Bayesian inversion framework for determining surface fluxes of trace species using an optimized grid. Geophys. Mod. Dev., 7, 2223-2242, doi:10.5194/gmd-7-2223-2014.
Thompson, R. L. et al. (2016), Methane fluxes in the high northern latitudes for 2005-2013 estimated using a Bayesian atmospheric inversion, Atmos. Chem. Phys., 17 (5), 3553-3572, doi:10.5194/acp-17-3553-2017.